What Does the Generative AI Ecosystem Refer To: As the field of artificial intelligence (AI) continues to expand, so does the complexity of its applications and components. Generative AI is one such element that has been gaining rapid traction in recent years. But what does the generative AI ecosystem refer to? In this section, we will explore the meaning of generative AI and its ecosystem to provide a clear understanding of the basics.
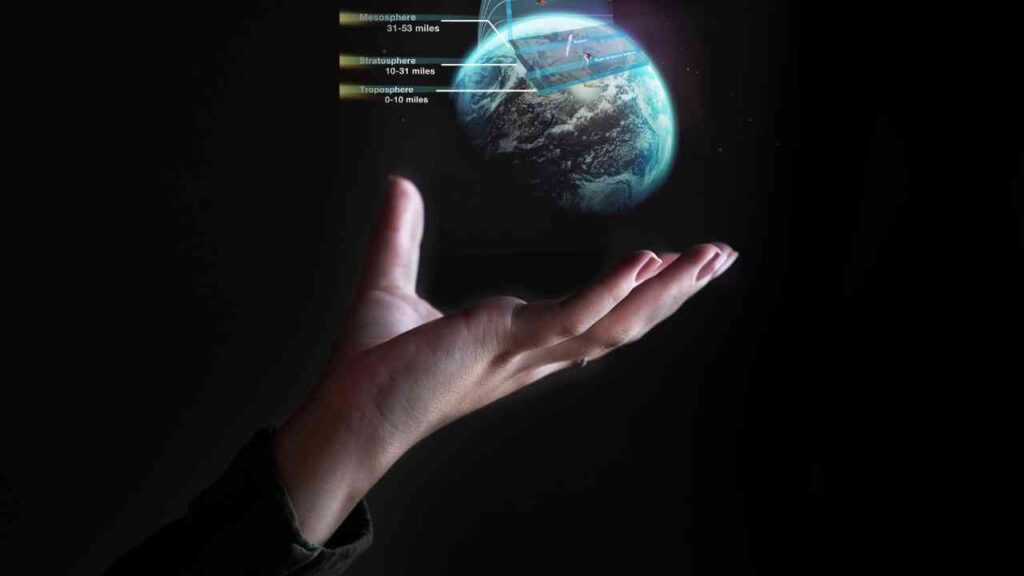
What Does the Generative AI Ecosystem Refer To: Key Takeaways
- Generative AI is an essential element of the larger AI ecosystem.
- The generative AI ecosystem is integral to the development and growth of AI technologies.
- Generative AI is used in various industries to drive innovation and solve complex problems.
- Understanding how generative AI works is essential to appreciate its potential and role in driving advancement.
- The generative AI ecosystem offers promising opportunities for businesses and healthcare organizations.
Understanding the Generative AI Ecosystem
As we discussed in the previous section, the generative AI ecosystem is a crucial aspect of the development and growth of AI technologies. In this section, we will take a closer look at the characteristics of this ecosystem, its importance, and the role of generative AI within it.
What Shall Be the Color of Auxiliary Lamps Installed at the Front of a Vehicle?
Characteristics of the Generative AI Ecosystem
The generative AI ecosystem comprises several key components, including algorithms, data, models, and hardware. These components work together in a highly complex and interconnected manner to drive innovation and advancement in AI technologies.
One of the key characteristics of the generative AI ecosystem is its ability to learn and adapt autonomously. Generative AI models can analyze vast amounts of data and use that information to improve their performance over time, without human intervention. This self-learning functionality is a crucial aspect of generative AI, making it highly effective in a wide variety of applications.
Another notable characteristic of the generative AI ecosystem is its scalability. AI technologies can be easily scaled up or down, depending on the needs of the application. This scalability is possible because generative AI models can be trained on large amounts of data, allowing them to handle complex tasks and processes
Importance of the Generative AI Ecosystem
The generative AI ecosystem plays a vital role in driving technological advancements in various industries, such as healthcare, finance, and entertainment. It enables businesses and organizations to analyze large amounts of data efficiently, resulting in better decision-making, improved productivity, and increased profits.
Moreover, the generative AI ecosystem offers businesses and healthcare organizations new opportunities for growth and development. Generative AI technologies can automate tasks, reduce costs, and enhance overall efficiency, empty up resources that can be used in more creative and innovative ways.
The Role of Generative AI in the Ecosystem
Generative AI plays a critical role in the ecosystem by providing the intelligence and adaptability necessary for driving innovation and overcoming complex challenges. Generative AI models can be used for various applications, such as image and video synthesis, music composition, and natural language processing.
Furthermore, generative AI models can adapt to new data and changing environments, making them highly versatile and effective in various industries. By incorporating generative AI into their operations, businesses and healthcare organizations can achieve better outcomes, increase productivity, and reduce costs.
Components of the Generative AI Ecosystem
Component | Description |
Data | Input data used for training and evaluation |
Algorithms | Mathematical models used to synthesize data |
Models | The generative AI models that process data and generate outputs |
Hardware | The physical devices used to run generative AI algorithms, models, and applications |
Applications of Generative AI
Generative AI has become a vital tool for businesses and healthcare providers to drive innovation and find solutions to complex problems. Let’s dive into some of the practical applications of generative AI and its impact.
Generative AI in Business
Generative AI is transforming the way businesses operate, from automating mundane tasks to delivering personalized experiences to customers. One significant application is in the field of marketing, where generative AI is used to create engaging content and ads. For instance, Netflix uses generative AI to create personalized movie trailers for its users based on their viewing history. The results speak for themselves, with an increase in user engagement and sign-ups.
Generative AI is also used in product design, where it helps in creating new and innovative designs faster. Siemens uses generative AI to optimize lightweight designs for a gas turbine blade, reducing the weight and improving efficiency.
Generative AI in Healthcare and Medicine
The healthcare industry has also started to adopt generative AI to improve patient outcomes and optimize care delivery. One application is the development of personalized treatment plans. For example, IBM Watson uses generative AI to analyze patients’ medical records and recommend personalized treatment plans for cancer patients.
These are just some examples of how generative AI is transforming industries and driving innovation to new heights.
How Generative AI Works
Generative AI is a complex field that involves the use of algorithms and processes to create new and original outputs. At its core, generative AI functions by exploring patterns within a given dataset and then using that knowledge to generate new information. This process involves several different techniques and methods, each with its own unique features and capabilities.
One of the most common techniques used in generative AI is known as generative adversarial networks (GANs). GANs involve the use of two neural networks, one of which generates new content and the other of which evaluates the authenticity of that content. This process results in a system that is capable of generating highly realistic images, texts, and sounds.
Another technique used in generative AI is reinforcement learning, which involves training a system to learn through positive and negative feedback. This technique has been used to great effect in applications such as robotics, where machines can learn to perform complex tasks through iterative trial and error.
Overall, the field of generative AI is constantly evolving, with researchers and scientists working to develop new and exciting ways to use these systems to generate creative and innovative solutions to complex problems. As we continue to explore the generative AI landscape, we can expect to see many exciting breakthroughs in the field.
In conclusion, we have explored the generative AI ecosystem and its significance in transforming technology. Through our discussion, we have defined and explained the meaning, characteristics, and importance of this ecosystem in advancing AI technologies. By examining the practical applications of generative AI, we have shown how it is being leveraged to solve complex problems in various industries, including business and healthcare.
Furthermore, we have explored the technical aspects of generative AI, delving into the algorithms and processes that power this technology. By understanding how this technology works, we can appreciate its potential and the role it plays in driving innovation and advancement in the field.
As we look to the future of generative AI, it is clear that this technology offers exciting opportunities for businesses and healthcare organizations. With its ability to generate new ideas and solutions, the generative AI ecosystem is poised to usher in a new era of possibilities.